Machine translation (MT) is the use of computer software to translate text or speech from one language to another. It automates the process of translation. At its most basic level, MT substitutes words in one language for words in another. By itself, that cannot produce a good translation as texts need to be seen as a whole. MT technology is being developed to overcome this barrier.
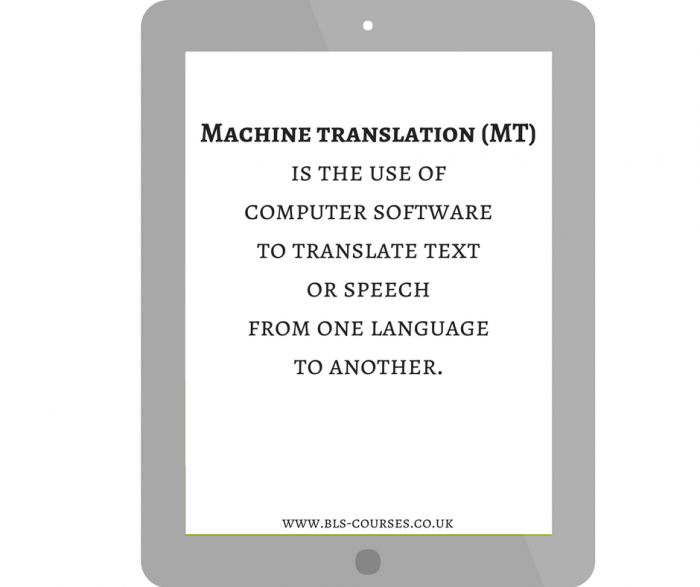
Advantages of Machine Translation
Machine translation, although not always perfectly accurate (see below), is faster and cheaper than human translation. This can meet the needs of businesses working in global markets.
Machine translation has also been hailed as a peace-keeping technological development. Its supporters think it can forge links between peoples and break down barriers. People who believe this accept that translation engines may never produce translations as good as human translations, but believe that they will be good enough to help people all over the world converse “as if language barriers never existed”.
Despite the un-idiomatic quality of some machine translation MT is sometimes acceptable to some audiences (and sometimes isn’t). It appears to depend on the attitude of the reader to language and whether it is a means of accessing information or expressing one’s identity as to whether they accept a machine-translated text (see for example the work of scholar Lynne Bowker on this subject).
Disadvantages of Machine Translation
There are still debates as to whether MT can or even should ever be a substitute for human translation. A machine-translated text may not be as idiomatic as a human translation and may not even be accurate, as a computer does not have a human brain. Translation is a creative process rather than just word-for-word substitution. Translators must look at a text as a whole, and know how words and phrases used may influence one another and what they mean in context. As well as having expertise in the language’s grammar and vocabulary, translators need to know about the culture and location the language originated in. This is not something that is easily replicated by computer algorithms.
There are also concerns that the translator profession may die out if MT is allowed to take over, or that MT will force translators to charge less for their work if they are to keep up with the market. The job of a translator could change radically and become unrecognisable – in the future there may only be post-editors who proofread machine translation outputs rather than full translators. Others argue that MT will boost the translation industry, as it will be called upon to improve the technology and fill the gaps left by imperfect automated translation with high quality non-automated translation.
How Machine Translation Works
There are different types of machine translation. Some software can be bought by companies who plan to use it regularly, and other software, available online and intended for one-off use by members of the general public, is free. There are generally two types of bought machine translation software: “customized” machine translation and “enterprise” machine translation. “Customized” machine translation involves “training” or adaptation of the translation software to recognize language belonging to a specific domain, industry or organization. It can be broken down into rule-based machine translation technology and statistical machine translation.
Rule-based machine translation technology uses vast databases of dictionaries and lists of language rules in both languages. The translation software uses the rules it “knows” to work out a translation that is likely to be correct based on the rules related to each word. Users of the software can improve the translation quality by adding their terminology into the translation memory. This means it can be customised by domain or profession, but it does not need to be as it works on language rules. This kind of software needs updating frequently but updates cost less than the initial purchase of the software. The quality of rule-based MT is consistent and predictable. It is not very fluent, though, meaning that it is not idiomatic. It also struggles with exceptions to grammatical rules.
Statistical machine translation, on the other hand, uses analysis of texts in the source language and target language to build translation models. This of course depends on what kinds of texts already exist. It still cannot achieve the creativity that a human brain can as it is only based on what the computer has “seen” before. A minimum of 2 million words for a specific domain and even more for general language are needed. Most companies, who would be using and “training” the translation software to write according to its house style, do not have enough existing texts in the required languages to build translation models. Statistical MT provides good quality when large numbers of usable texts are available. The translation is fluent, meaning it reads well and therefore meets user expectations. However, statistical models do not know about grammar. They can handle exceptions to rules, though, unlike rule-based translation technology.
These two models show that “customised” solutions that “train” their software are only as good as the data provided. Nevertheless, improved output quality can be achieved by human intervention: for example, some systems are able to translate more accurately if the source text (the text being translated) is made easier for a computer to translate (removal of idioms, culture-specific names/references). Otherwise, “post-editing” (improving the machine translation by having a human proofreader check through it) can be used.
“Enterprise” Machine Translation is the stuff of “next generation” of “augmented” machine translation engines. These employ sophisticated technology and localisation techniques to reproduce personalised, customised terminology, styling and formatting across languages. It is fast and can produce high volume content and real-time multilingual communication, which is what global businesses want.
“Generic” machine translation is instead a ‘one size-fits-all’ solution used by search engines that translate text. Used by individual internet users for ad hoc translations of short texts, “Generic MT” is less accurate than “customized” machine translation. This model of machine translation “throws […] data at its engines in hope for them to become better with time”. An example of this is Skype translator, which is already up and running but is not perfect as it still needs to “learn” how people speak. Skype would argue that this is more than just “throwing data at its engine” as it learns from structured communication such as conversations.
Have Your Say
This is definitely a domain that sparks a lot of interest and debate. What do you think? Let us know on our Facebook page!
Written by Suzannah Young